Artificial intelligence
Incorpora video
Artificial intelligence
Advances in artificial intelligence can be seen as a drop in the cost of prediction. As the cost of prediction falls, simple economics says that we will engage in more prediction and that the complements to prediction become more valuable. This presentation uncovers the implications of cheap prediction for organizations and for society.
i don't know i'm a researcher at the bruno casa foundation i'm here today to introduce our guest professor avi goldfabb professor avi golfab is an economist he's a helizo professor at the marketing of at the rodman school of management university of toronto he has also worked at the national bureau of economic research and he also works in a center within the toronto university act as an incubator of a startup sub in the field of ai the center is called creative destruction lab and is experienced with startups has led him to write a very interesting book that was recently published entitled the prediction machines and the main topic will be dealt with by professor goldfarb in his presentation i guess but the main point with the ai is the ability to make predictions predictions not doesn't mean magic predictions about rather than rather reducing uncertainty machine prediction or automatic prediction becomes therefore cheaper what's interesting about this book in my opinion and i do hope a professor goldfarber will help us understanding more about these items is that by reducing the cost of prediction we do not destroy jobs in other words we would not replace the people who are in charge with predictions but rather by shifting the accent on judgment the business model in many companies may change and this will lead to rethink in the business model within companies and ai not necessarily will contribute to job destruction but rather enhance some other aspects of the value chain therefore creating more jobs and more opportunities that said i would like to hand you over to professor avi goldman thank you very much hi okay so uh this is this is based on on the book and on work and i work that i've done with uh jay agarwal and joshua gans and underlying all of this is this excitement around this technology we have this sense that ai is transformative and it may really even change everything and at the same time there's a little bit of anxiety so wait if the machine's intelligent that what about me what does that mean for us humans if we have these intelligent machines intelligence was supposed to be the thing that distinguished us from everything else yes we've accepted the machines are stronger than us but maybe we're not quite ready to accept that machines might be smarter than us now underlying all this is a lot of confusion about what's really going on what are we talking about when we're talking about artificial intelligence the optimistic point of view in artificial intelligence is we're going to have something like c-3po from star wars c-3po is a robot that can do everything a human can do it's fundamentally intelligent with one exception c-3po when given instructions by a human listens humans aren't as good as that but otherwise we have a machine that's really human-like the alternative type of ai we see in the popular press and in science fiction is skynet from the terminator or the results of the movie the matrix where you have a machine that's truly intelligent and they realize they don't need to listen to humans anymore and they take over the world that's not so good now to be honest i do not think the idea of truly intelligent machines is crazy but i think it's important to recognize it is nothing to do with the technology that's gathered uh that's generated the excitement that makes us talk about ai in 2018. the reason we're talking about ai in 2018 is because of advances in a particular type of computer science called machine learning and that is prediction technology and so when you hear ai in the context of business and government today don't think intelligent machines think prediction technology what's happened is prediction has gotten much better faster and cheaper than it ever has before now that might sound mundane but it turns out cheap prediction better prediction can be pretty transformative to get your head around that i think it's worth jumping back a technology this is uh the technology that we made our career study until recently and it was the internet looking around the room it looks like maybe half of you remember 1995. 1995 was a very exciting year in technology little history lessons 1995 was the year that the last public aspects of the nsf net were privatized it was the year that netscape had their ipo they were valued at over a billion dollars with no profit and it was the year of bill gates internet tidal wave email where he said the future of our company is the internet and if that was about microsoft at the time that meant the future of software in the future of computing was going to be the internet okay so a little bit of emphasis okay um now over time that hype get building in 1996 1997 1998 people stopped talking about this as a new technology and they started to call it a new economy the changes seemed so transformative that in the press you were reading we don't need to listen to our old rules anymore we can throw out our textbooks this is transformative this is fundamentally new now there was one group of people who said it's not a new economy that was economists right we said no no no it's the same old economy you can understand it in a pretty straightforward way some things have gotten cheaper and as economists we know when certain things get cheap we do more of it and that can be transformative in the context of the internet thinking about it as search is cheaper communication is cheaper and replication is cheaper and once we understand that we can understand the consequences for example what does cheap replication mean it means that copyright and privacy are going to be a big deal a much bigger deal than when replicating something when copying something was more expensive once we understand what's changed we can understand the consequences of a technology let's back up another generation and think about computers think about the semiconductor what does your computer do at the end of the day you think about it you have these powerful computers laptops in your pockets what do they do they do one thing and as economists we like to think about it again as a drop in the cost of something so what does your computer do it makes something cheaper only one thing arithmetic your computer does arithmetic that's it that's all it does but it turns out when arithmetic is cheap enough we find all sorts of applications of arithmetic that we might not have dreamed of we all understand this this is economics 101 this is demand curve slope downward when something is cheap we do more of it well demand curve slope downward in the context of arithmetic and when the price fell so dramatically the uses for arithmetic exploded but the first uses for machine arithmetic were the exact same as the first uses for human arrays uh in the wars you know world war ii and shortly after we had cannons and they shot cannonballs and it turns out it's a pretty difficult arithmetic problem to figure out where those cannonballs are going to land how do you solve that problem with arithmetic we had teams of humans who in the united states their job was called computer the movie hidden figures was about these humans whose job was to do that arithmetic then computers came along machine computing and the arithmetic was cheaper that way and so the machine computers replaced the human computer then everything got a little bit cheap a little bit cheaper and it found another classic arithmetic problem accountants used to spend their lives adding and subtracting that's what they did if you look at what an accountant's job was in the 1940s and 1950s what they spent their hours doing it was literally adding up columns of numbers accountants don't do that anymore machines do that because machine arithmetic is so much cheaper than human right now this is going to give us a sense of where the hope is because it turns out there's still plenty of accountants even though they don't do arithmetic why because the people who were best at doing arithmetic turned out to be the people who are best positioned to figure out how to use arithmetic to benefit the company or to figure out taxes or to think strategically so cheap arithmetic yes it meant accountants weren't doing arithmetic anymore but there's still plenty of things for accountants to do but then arithmetic started getting even cheaper and we started to find a whole bunch of applications for arithmetic that most of us never dreamed arithmetic with the possible exception of adelevlace about 200 years ago for the rest of us we never thought about games and mail and music and pictures as arithmetic problems we thought of them as chemical problems or communication problems we didn't think about them as math but it turns out when arithmetic is cheap enough all these things are arithmetic again this is demand curve slope downwards this is when something becomes cheap we do more of it and at first we do more of it in the same way and then over time if it's a transformative enough change we can find new applications new uses that we never dreamed of before so that gets us to this this is a representation of convolutional neural net this is one of the technologies underlying the current excitement around ai what is this this is prediction technology what do i mean by prediction i mean prediction in the statistical sense prediction using information you have to fill in information you don't have prediction can be about the future but it doesn't have to be about the future it can be about the present or the past it's just filling in missing information you know something and you want to know something else one of you and you use the data you have to fill in the data you don't have prediction turns out to be really important and when we think about these changes we should think about them as a drop in the cost of prediction same graph different axes same idea the economics are the same demand curve slope downward prediction gets cheap we're going to do more and more and more and just like with arithmetic the first applications of machine prediction are just like the predictions we used to do before you walk into a bank and you want a loan and the bank's got to figure out the bank's got to predict whether you're going to default on that loan or pay them back that is a prediction problem that's hundreds if not thousands of years old and now increasingly we're using machine learning to make those predictions similarly the insurance industry you want insurance has the insurance company figure out how to price that risk they make a prediction on your likelihood of defaults and how big that claim is going to be insurance is fundamentally a prediction problem and increasingly again we're using machine learning we're using ai to bill in that missing information to help with that prediction but as prediction gets cheaper we're finding more and more and more applications for machine prediction that we might not have thought of as prediction problems before just like pictures are arithmetic we figured out that medical diagnosis is a prediction problem what does your doctor do they take in information about your symptoms and fill in the missing information about the cause of those symptoms object classification object recognition facial recognition is a prediction problem how do i know that shane sitting right there well my eyes take in signals of who that is they say okay they fill in a context and a name and fill in the missing information of who that is and and why i know him from the past now as prediction gets cheaper we really can start to think about reformulating problems that don't seem anything like prediction into prediction problems autonomous driving or driving more generally has been reformulated into a prediction problem there's the obvious prediction problem which is that there's all those other crazy drivers out there and you need to predict what they're doing but really the core insight driving the recent advances in autonomous vehicles is the insight that we don't really need to tell the car what to do we just need to tell the car to predict what a good human driver would do essentially you have the car sitting on the human shoulder watching millions and millions of miles of driving not literally sitting on the shoulder but he's sensors around the car and feeling and saying okay here's the situation what does the human do with this situation and it just needs to predict what the human would do instead of have a whole bunch of if then statements to understand what's going on and once you understand that you understand that a lot of the action is about filling in this missing information and about the creativity of reformulating old problems into prediction problems you can see there's a lot of opportunities here and we're only a few years in i don't know what's coming next but i'm excited about what it might be now why is prediction valuable right there's lots of technologies why uh do we think this one is particularly interesting and important relative to many of the other technologies that have risen over the past decade prediction is useful because it's an input into decision making and decision making is everywhere right you make big decisions what job should i take when should i retire should i marry should i have kids can you make smaller decisions should i scratch my face should i write that down these sorts of things you make big and small decisions all the time and each of those decisions has a bit of prediction in the background but the important thing to remember is prediction is not decision making there's all sorts of other aspects of decision making that aren't prediction how do we unpack that well and why does that matter well let's think back to econ 101. so when the price of coffee falls we buy more coffee right that's demand curve slope downward you learn that on the first day of your first economics class second point when the price of coffee falls we buy less tea those are substitutes when the price of machine prediction falls the aspects of your job that our prediction are going to decrease are going to become less valuable machine prediction becomes better human prediction becomes less valuable they're substitutes coffee becomes cheaper we buy less tea the third thing that you might remember from econ 101 hopefully more of any constitutional audience uh is that when the price of coffee falls we buy more cream and sugar when the price of coffee falls the compliments to coffee become more valuable and so the question you need to ask with respect to machine prediction is what are the cream and sugar for prediction what becomes more valuable as prediction becomes cheap and since we know that prediction is an element of decision making what we can do is unpack decision making so here's our representation of what goes into a decision broadly speaking you have your prediction at the center because that's what the folks are called doesn't necessarily have to be at the center but that's what we're focusing on different kinds of data so we've heard this data is the new oil data is exciting um you have an action which is there's no point in making decision if can't do anything with it so the ownership of the customer relationship the ownership of the action is valuable and then finally uh what meant what i think is maybe the most interesting part is this idea of judgment what do we mean by judgment judgment is evaluation judgment is figuring out what the payoffs are any particular action means knowing which predictions to make and knowing what to do with those predictions once unpack that i like to use another movie example which is the movie irobot anyone see this movie it wasn't a great movie um but there's one scene in that movie that i find particularly useful for understanding this idea i really love that scene flashback scene and um so will smith is a star and he's driving and he's in a car with a little girl and for some reason that he has to swerve and the car goes over a bridge and it's sticking into a river and it looks pretty clear they're both going to drown in the river and then a robot comes along and saves will smith and not the little girl and the question is why does the robot do that and will smith goes back and he unpacks it what he figured out is the robot predicted that he had a 45 chance of survival and the girl only had an 11 chance of survival so the robot saved will smith and not the girl because he had the robot predicted that will smith had a higher chance of survival will smith thinks that that was the wrong decision in the movie he says 11 was more than enough the robot should have saved the girl and a human would have known what he said that's judgment judgment is knowing the relative payoff in addition to that prediction the prediction's not good enough to make a decision you also have to assess is that little girl's life really worth four times will smith's life and it might be and maybe we as humans would have made that decision but then at some point her probability of survival would be low enough whether it's five percent or one percent or point one percent that will smith or perhaps any human would say you know what the right decision is to save wilson when we're making human decisions and we make decisions because we can mix prediction and judgment we often don't have to be so explicit about what the payoffs are now we teach you're supposed to be you know take a decision theory class uh you learn that you're supposed to be that clear and specific but for the most part we mix these things up and we don't bother but when you're working with a prediction machine you have to invest and spend time in figuring out what matters what you care about as an individual and as an organization and that's going to be a key skill if not the key skill in working with these machines okay so so judgment is uh understanding the payoffs of any particular action so i was just giving the slowdown signal okay um so tools what do we do with this we have this prediction technology why is it useful how is it actually used within an organization and so the headline looks something like this the headline is goldman sachs moves to automation and as they move to automation they used to have 600 traders as far as we can tell by the numbers they may even employ more people than they did back when they had 600 traders the key point is what you do is you unpack uh the workflow figure out within a workflow what are the things that we can put a prediction machine in what are the things that can be automated and when you do those things more efficiently you actually might need more people in other aspects of the workflow for example what they do if they're figuring out well can we automate can we make our ipo process how do we do initial public offerings how do they do that he said well you know what there's 146 steps in an ipo and then they might say you know what step number 132 that's right that's a prediction problem we can insert a prediction machine and so the humans who are doing step 132 would be doing step 132 but that may mean that steps 131 and 133 uh become more important more efficient more valuable and so the net effect on employment within the company is ambiguous it just depends it's not always going to be positive but it's not always going to be negative now sometimes these changes sometimes the drop in the cost of something changes the structure of an industry it can change the strategy you think about search who did research in 1990 who owned the idea of if somebody wanted to look something up where did they go they went to the library in 1990 if you wanted to look something up you went and asked a librarian but if you asked a librarian in 1990 do you think library science is going to be one of the biggest businesses of the next decade every librarian you talked to would have thought you were crazy but it turned out when search became cheap enough that search moved beyond us it moved beyond researchers to being something that was invaluable to the general population the number of searches that occurred the number of people said you know what i want to know the answer to that um and i want a you know an electronic tool i want a useful tool that can get me there was really high when search became cheap a whole industry was born and it combined what might have been the yellow pages and the white pages and libraries and eight other search technologies into this one really efficient business so what does it mean to really focus on on ai so here's here's the ceo of google he said you know google used to be mobile first now we're going to be ai first and a lot of that sounds like okay business speak in some sense but what does it really mean let's unpack what mobile first means what mobile first means is that we're going to make the mobile site better at the expense of the website or potentially even at the expense of any other uh customer touch points we the mobile site is the thing that we're going to focus on most mobile first means other things second now when they said they're going to go to ai first ask their director a director of research there what does ai first mean what he said was ai first means we're going to be really good at ai by that means well with search because you get 10 links or 20 links we can be wrong sometimes and people just look down to the next one but if we're going to be an ai focused company if we're going to provide an assistant then an assistant making a travel reservation for you can't be wrong twenty percent of the time it can't even be wrong two percent of the time you gotta be really really accurate ai first means accurate and this headline gives you a sense of what was really going on what does ai first mean ai first means that the ai team sat next to the boss the ai team in casual got to have casual conversations with the boss as they're sitting for lunch as they're going to the elevator etc more importantly to really get the idea what ai first meant is that something else came second there were people who used to be next to the boss who aren't anymore that's what ai first means it means making a choice to prioritize this initiative over other initiatives prioritize learning uh and gathering data potentially at the expense of the short-term customer experience prioritize the ai team at the expense of whatever team used to and imagine what could happen as the prediction technology improves think about all the compromises you do because of uncertainty because of bad prediction with the small scale you think about an airport lounge you go to the airport and if you fly a lot they have this wonderful lounge there or okay maybe wonderful a bit of a stretch but you go and it's more comfortable than the regular place you can go and sit while you wait for your flight why does the airport lounge exist it only exists because of bad prediction right if you knew how long it was going to take to get to the airport and how long it would take to get through security you would never use an airport that infrastructure is entirely a compromise because of bad prediction think about other compromises think of amazon we think of amazon as an ai leader in many ways and so they make predictions about the things you might want to buy and they have millions and millions of products and they make predictions about what you're going to want to buy they put it on the page maybe recommend it for you and from my experience maybe they're right one out of every 20 times i know what the real numbers are but let's say they recommend 20 products i'm going to buy one of them that's not bad even they have millions of options i don't want you know i don't want most of the catalog but what it means for their business is their business is the same business that catalog companies have been in for over 100 years they're in the same business that sears was with the sears catalog and what does that mean there's a catalog you shop through it you look at the catalog you choose what you want they send that to their warehouse and the warehouse ships it to your door the shopping and shipping business model why because of uncertainty how do we think about this let's turn up our prediction dial let's turn it up um i don't know where but whether it's gonna be ten percent or fifty percent or seventy percent or ninety percent at some point they won't have to wait for you to order at some point their prediction machine is gonna be good enough that it's worth it for them to ship the items right to your door you open the box at your door you take what you want you leave the rest and then they build an infrastructure for developing those returns now to be honest i have no idea if this is really going to happen uh but what i do know is that they've thought about it they have a patent took it out a few years ago anticipatory shipping so this idea that as prediction gets better we can overcome some of the compromises we make some of the ways we serve our customers worse and serve them better because of better prediction about the ai industry the hr industry you're in hr human resources you might think you know what i'm in an industry where i deal with humans i don't have to worry about ai well not really a good chunk of what people do in human resources is prediction they predict hiring is all about predicting which humans are going to work well in your organization so that's inherently a prediction problem and a lot of what human resources people do with their time is prediction so it's already happening that resume screening or thousands of applicants for a job that initial screen is increasingly done by machines but it could go further it could get to the point that you don't even need to post a job at all you don't have to worry about that uncertainty about who's going to apply who the right person is whether they're out there what you could do with the right data in a good enough prediction machine is identify the person you want to hire and say you know what i want to hire you and your prediction is good enough that you know that they're going to respond to you and say yes okay now again these are thought experiments exactly which ones are going to happen who knows but the underlying point is we make lots of compromises in our workflows in our jobs in our organizations because of uncertainty in a prediction machine machine that reduces uncertainty is going to help and understanding that in terms of where you work and what you do is a statement about thinking through what it means for that dial to turn and hypothesis about how quickly when i see what's actually going on now i have to admit i'm a little underwhelmed those amazon predictions are fine many of you will probably think that they're not really that good they seem to just recommend the thing that i looked at and didn't buy because i didn't want them and maybe a few others it just doesn't seem that transformative technology or siri or amazon's alexa they work and they're kind of cool but they're frustrating and imperfect and there's all sorts of flaws underlying the technology how do we how do we make sense of that while at the same time we see investment in this industry going through the roof we see governments around the world saying this is an important technology potentially the important technology we need a national strategy around it france is a national strategy around ai the united states has had their various national strategies around ai they don't know if italy does no used to um you know this man whether he's a national strategy around ai or not i don't know but he's very ominously said whoever controls ai will control the world china has invested billions and billions and billions in billions and saying they're going to be number one in ai by 2030. and then we have google and microsoft and others who said their focus is ai they're ai first how do we make sense of all that well that's that's a statement about different theses on how fast right now the dial is pretty low right now this is conjecture in the sense that these are prediction machines we think prediction is going to get much cheaper and if prediction gets much cheaper extraordinary things will happen the the dissonance between what's happening now and what people are projecting will happen in the future is a statement about how fast we're going to get to uh from where we are now to really really cheap prediction how fast thank you very much it was very very fast and much a lot of time for questions thank you for watching so thank you for writing this book and i i think for the general interest it's worthwhile to spend some minutes on performance versus privacy in europe we had gdpr and i think we had been lucky because of the facebook cambridge analytica scandal that made many u.s companies decide to embrace gdpr and as the main competitor in this field is china then uh could be that europe and usa join force together in a alternative way then china is using i know china is has a lot of advantages because they don't care on privacy and so on so they have more data the government is very cooperative with companies and this is not so in europe and usa so what do you think about this um i actually think a lot about this um so um oh here thank you all right so thinking about privacy um so europe's had stricter privacy regulations than the us and china for a while and um i have some research from about a decade ago that shows the previous generation of european privacy regulation uh seriously affected the advertising supported software industry here okay and so um you know the the evidence just says that advertising doesn't work as well in europe as it does in the united states um the conjecture is therefore you would expect the biggest advertising supporting companies in the world google facebook etc to not be in europe um now um how does how do we think about this going forward with gdpr um and a variety of things so there's a few things to think about one is um if access to data is a strategic advantage at the national level are we going to start thinking about privacy as something that belongs in trade agreements so dan treffler and i have a uh paper coming out or a thought piece coming out for a world trade for the world trade organization where we think just like environmental regulation is now part of trade agreements um so that we avoid a race to the bottom you would expect if we want to protect privacy still keep some advantage that uh data use and data restrictions may be part of trade agreements going forward so that's a somewhat of a pessimistic potentially point of view in the sense that yes i do think that at the national level privacy regulation hurts innovation i want to add a very important caveat to that which is that at the company level at the strategy level i do not think that's true having individual companies choose uh what they think the right level of privacy is um there's lots of evidence that companies that are privacy respecting do very well and so my statement about um the trade-off between uh privacy and innovation is a statement about regulation and not a statement about strategy now another important subtlety here is um that it's not obvious that gdpr or any of these regulations are really restricting the amount of data that big companies and so um you know the the regulations around gdpr at least as i understand them largely are things that with resources and consumer trust a company can overcome and so there's been some preliminary evidence already and certainly some conjecture and hypotheses that google isn't going to be that hurt by this uh in europe or you know if it adopts equipment policies in the u.s and the extent that anyone is hurt it's going to be companies that don't exist yet that would try to get traction and don't have that consumer fund so again this is going to be a challenge and thinking about it's still a trade-off that's a trade-off between privacy and um and market power essentially which now another import so there's two important caveats to all this pessimism important caveat number one is what i just said that from a strategic point of view an individual company privacy respect respect often makes a lot of sense and the other important caveat is that look if privacy is a right that's only meaningful if it means we're trading things off for it there's no point in thinking about a right that we would want anyway that helps everybody it's a right by definition because it's costly to have that right and so if you think that we have a right to privacy it should come as no surprise that that has some costs that's that's that's why it's important that's why we need to write it down that's why we need to value it why it needs to be if it has no cost that we don't need to bother because everyone will do it anyway and so um so to sum up the regulatory environment with respect to privacy in china gives china an advantage relative to the us and the regulatory environment in the u.s gives the u.s an advantage relative to europe for sure um but that doesn't mean that it's not a good idea um in the context of thinking about what a right means what it means what human rights are all about other questions okay thanks thanks for your presentation very brilliant and informative one question i had was learning uh from facts and mistakes it's not the same across a variety of circumstances so in particular there are examples that can be made in which i guess the speed of improvement even of machines if there are so many uh crazy drivers around then a machine perhaps maybe actually misled by seeing uh so many rapid repetitions uh from so can we sort of rank the uh in your views the activities from which we can learn i've seen that there was this report mckinsey global institute years ago in which they were saying what was automatically more likely to be automatically or not so something like this related to what you are saying and ability to predict uh and the speed of learning in this area so so there's there's been a bunch of studies that look at the tasks that are involved with the job and then assert these tasks seem to be automatable and those tasks aren't and therefore this percentage of jobs is automatable so there's this uh freyan osborne paper that was um that had a huge impact that said 47 of all jobs are going to be automated i have no nothing wrong per se of what they with what they did in the sense that if you follow the steps that they made they get to 47 and that is correct but there's a few there's some really important caveats there caveat number one is they didn't put a timeline on it and if you you talk to them and read what they described actually you know in that they were pretty clear that this is a 50 to 100 year timeline this isn't a five-year timeline and the world changes a lot for 5100 years and i would guess most aspects of my job today most of the things i spend my time on even as a professor which is a famously not changing job are different from what my peers you know from what my uh potential senior colleagues would have done 50 years or 100 years and so that gives you a sense of where some challenges are now in terms of thinking about learning per se that gets really interesting uh in a few ways one is learning is costly right so we know that as humans we spend years and years and years learning we take time off from work where we're not essentially being explicitly productive in order to learn more things it's a costly endeavor it's a costly activity it takes time it takes effort it takes money computers are no different it's costly for computers to learn and just like um and we're going to take different risks based on context for how much we want to wait for somebody to learn what do i mean by that so um you're going to hire somebody to be a cashier at mcdonald's okay you need to train them you don't need to train them for very much maybe it's an hour or two or a day and after that you'll throw them into the workforce you'll have them start working serving people and you know what they're gonna make mistakes they're gonna be a lot worse than the person who's been working at that counter for two years but it just doesn't really matter because the stakes are pretty low in contrast airline pilots commercial airline pilots right we don't give them a day or two of training before we say okay go fly a plane with 300 people in it there's a requirement of a lot of learning because the mistakes of doing something wrong that insight that intuition also applies to machines so if the stakes are pretty low then it won't take long to get the machine out into the field and once the machine's out in the field it can learn really quickly just like once the mcdonald's employee has been trained and is out working at the counter they're gonna learn very quickly how it works much more efficiently than in the training same thing with the machine once the you know if you had a self-driving car and you put it out in the field it's going to learn from mistakes very quickly the problem is those mistakes are really costly in contrast you know if any of you have gmail google's email and it fills in uh the text it fills in your email and says oh this is i think how you're gonna respond the consequences of it being wrong are pretty much zero it may be a little annoying for you but otherwise they're pretty much zero so that they could roll that out when it was pretty bad and they're only right three percent of the time that's good enough let's roll it out see what happens your autonomous vehicle was only right three percent of the time we would have a real problem and so you know we think about learning it's there's two aspects to it there's a statistical point which is that you need a lot of data but there's a strategic point as well which is you have to decide when it's worth putting your machine out in the wild where it can start to learn quickly and you can start to make money off it or at least benefit from it in some way at this cost of um thinking through what happens if something goes wrong does it really matter um thank you for your presentation and um i was wondering that you know in china they have access to all the data of their citizens but what about the u.s you know that all the data from this the people like all over the world would be like manipulated and controlled by few companies like google amazon facebook what would be the implications of this oh okay give me a left turn i thought you were going somewhere and you went and um that you're gonna talk about geopolitics and you end up in market power um so so we'll talk about market power that's where you ended um the first thing to remember about market power with respect to data is there are in a static literal sense or a technical sense decreasing returns to scale and data there are not increasing returns to scale and data and and if you've taken any stats that's obvious right your predictions improve in the square root of n what does that mean it means your 10th observation is more valuable than your 100 which is more valuable than your thousandth which is more valuable than your million so in a technical sense we have decreasing returns to scale and data not increasing returns and so in a technical sense in a static sense you have uh what makes your predictions better that we don't have economies of scale and data that doesn't mean there isn't a story to tell so the the story about a handful of firms dominating has to rely on some other economics there's a few potential stories there one is it has nothing to do with data and it's all about platforms and platform competition this is not an ai story in a data story this is about uh direct and indirect network externalities it's something to think about and worry about but it's not it's not really about the ai technologies about the previous generation or maybe even the generation before that of it story number two is in a yes there are diseconomies of scale and data decreasing returns to scale and data in a static sense but if but a dynamic sensor might not the story number two is if being a little bit better than your competitor means you get all the customers that means you can have a small lead in data that can turn into through a feedback loop into a bigger and bigger and bigger for that to happen something uh you need something a little bit subtle which is that the the other company your competitors can't just undercut you in price because of your leaded data so there we have to have some lower bound or some essentially bertrand competition really fears competition and quality and really in no other dimension that you can think about in and to understand if that would happen in the context of data you really need to think carefully about industry by industry whether that's likely to be true it's hard to think about it in industries where prices are really important the third um potential story to overcome this uh decreasing returns to scale and data has to do with endogenous sun costs around building a data science infrastructure and data science teams and so there it's again not about the technical point of decreasing returns to scale and data because there's or increasing return sales because there aren't there's decreasing returns to scale and data but if we think about sutton and r d and advertising we can imagine the same investment in terms of um in terms of data and data science and data infrastructure that uh we just keep making you know the companies that have a lead keep investing in it to make it better and better and better so that we haven't dodged into some costs and we end up with a small number of players not just some cost stories usually don't lead to monopoly but they do lead to a small number of players so those are the so the the first thing to remember is in a technical sense decreasing returns to scale and data so the story doesn't actually doesn't gel at first and then you'd have to layer on some other economics to to get to a point where we'd worry about age trust in dominant firms any other questions i have a question for the other one i was very intrigued about your example of the coffee and the sugar and the tea was i wondering what i'm wondering was if we want to to understand what our skills we need for the future of jobs uh we probably would like to understand what does need for us to be the sugar what is what is the sugar in this case in your book you taught a lot of you talk a lot about judgment as a way of being the sure so the real human right uh so my first part of the question is uh what what are the skills in order to be good at judgment and the second part of the question is that i think that actually there is another thing in between the prediction and the judgment which is the assessing of the prediction so you have to understand how much you believe in the prediction of course you can have a prediction that is accurate 99 but still there is one percent probability of being wrong so so just to clarify so that issue is judgment that issue is knowing what to do with the false positive and false negative so oh you're 99 right one percent wrong um is is a statement about having knowing what the payoffs are down the whole decision one part is knowing the the payload the other is understanding uh what is likely i mean can i believe you in the prediction sure uh because i think that uh yeah one is in machine learning especially the new machine learning the deep learning actually the decision is uh obscure is obfuscated you don't have a clear relationship between the parameters and the outcome okay so um so there's a few some interesting subtleties there so the first um first thing is should we try we have a prediction machine should we trust the person and um in some sense if we've learned anything from daniel kahneman and dan ariely and others is when humans decide to not trust the prediction things go badly in a general sense um so you know so if we have a prediction machine and we ignore it generally it's our egos that make us ignore it and we're just wrong and so what really matters is to have uh to understand what probabilities mean and then to think through the payoffs which is judgment um with one important subtlety and so when you say what are the jobs in terms of the future you can think about three categories of jobs there's one category of jobs which is the people who build and understand the prediction machines so these are science technology computer science math type jobs and these are you really need to know what it means to get a prediction and uh and in particular as economists we know the biggest failure in a prediction machine is going to be with respect to causal inference and so we need to understand when uh causal inference fails which means we actually don't have data on a counter factual we don't know what's happening and so we think we have a good prediction but it's actually not a good prediction so you need people who understand the machines that's that's just set of jobs number one jobs number two is you need people who tell the machines what to do okay so this is the judgment this is what do we care about as a society what do we care about as an organization what do we care about as people what are those valuations so that's essentially a set of jobs around judgment the people who make the most money in that are also going to understand the machines right because those things someone go together they don't have to know how to code them but they need to understand what they're useful for and so there's a set of jobs around building machines there's a set of jobs around judgment and codifying um values which maybe optimistically in the long run is a set of jobs for humanities rather than for science or social science understanding what values are um and then the final set of jobs are actually around the action so not really around judgment at all which is that some things are just better if done by a human the most obvious one of these to me is professional sports right you know a you know a race between robots or a football game between robots does not sound very exciting uh some there's something about having a human doing that that creates the opportunity now admittedly there aren't that many jobs for professional athletes but entertainment generally is an industry where there's going to be a future for you and so we have these three categories which are um which are you know coders and people who build the machines engineers we have people who know what we value on judgment and then we have people who um own the actual action because uh because by their very nature of being human uh other humans value that's why i think about that we have time for one or two more now will artificial intelligence learn to judge so slowly and gradually will ai be able to do what a human being can do there is a provided certain judgment will ai be able to do this or will it not with time and speak slowly please uh the question as i understood it was will the machines learn judgment and that's a fantastic question and we have a whole chapter in the book dedicated to that so the question which is that well or more precisely can a machine predict judgment and the answer is yes given the right kind of data a machine can over time predict judgment and so now that that isn't quite as hopeless as it sounds because the limit of a prediction machine is on data and so as long as there are limitations to the data that the machine can get there's going to be limitations to the ability of the machine to predict judgment and so in some sense a lot of what the machines are doing is predicting judgment when you think about that amazon ai that's predicting what you want what's it doing it's predicting your values for the products they have so they're predicting your judgment about product guidance yes predicting judgment is you know is a thing it's something to think about it's something uh that we would expect to happen over time but the limits of that are important and this this actually is related to the previous question which is the limits of prediction machines are uh based on lack of data and there's two ways you can think about lack of data there's lack of data in the context of rare events some things just don't happen very often it's hard to predict earthquakes because we just don't have enough data on earthquakes it's hard to predict the consequences of elections because they only happen periodically and each time they happen the world has changed so much that we really don't have a lot of useful data for predicting that so prediction machines are going to be bad at rare events but the other case that's a little less intuitive in thinking about missing data is with respect to causal inputs okay so you guys came to this wonderful conference on economics and technology and jobs and let's say you know you go home after this and whatever your aspirations and careers uh goals are with respect to technology and the economy and jobs you become spectacularly successful so if you want to go into a business you build an ai business and become billionaire if you want to help advise government you help advise your government and your government becomes the best government in the world by far and everybody who's doing this comes to italy okay whatever spectacularly successful can we say that you coming to this conference made a difference we can't because we don't know what would have happened had you not shown up and so what that means is we don't know the counter factor we lack data and so you should think about that idea the count of causal inference the reason prediction machines fail at causal inference the reason they can't figure out hey was it a good idea to come here is because we don't have data on the counter factor it's a statement about missing data so prediction machines break down without data that includes rare events but it also includes causation and so unless you're in a situation where you can simulate or run experiments in order to get a causation and machines in principle could do that with in cases where there's lots of opportunities to collect data then the prediction machines are going to fail and so yes uh machines can predict judgment but only within these limitations of the fact that these machines need data in order to predict that's a great question on that note uh maybe we're done or do we talk to one more well if there is another brief question we can we can take it somebody else okay so a question about automation and politics we have a prime brand new prime minister we have an under secretary to to the premier so to the prime minister i was wondering whether you think that the machine could be could substitute at least the under secretary because the under sector somehow is let's say collecting the information and provide instructing some of the procedures but then maybe we need a prime minister but at least a machine could perhaps do a better job than an informed uh machine could do a better job wow so so we need to figure out what we value so even in the extreme limit where we have machines doing all our predictions for us we need to figure out what we value and maybe that can be embodied in a particular individual as the prime minister but historically when we think about the bureaucracy in governments they don't just provide predictions they also provide some value or in some sense of what society as it overall values and fines and so so i think the under secretary's job is safe for now and um you know the political economy of the consequences of this automation are a whole nother thank you very much thank you very much professor for being here thank you goodbye you
Contenuti correlati
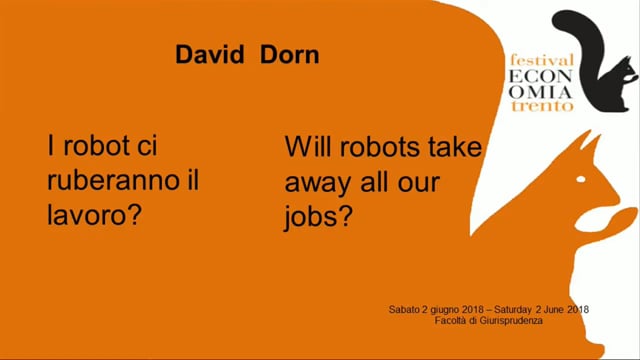
Will robots take away all our jobs?
Edizione 2018
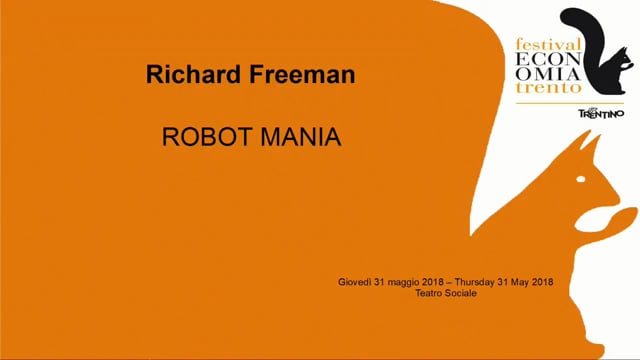
Robot mania
Edizione 2018
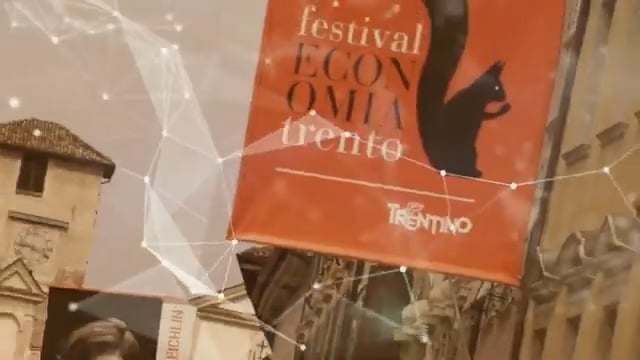
So will the recovery be for everyone? - Visions
Edizione 2021
Tematica simile
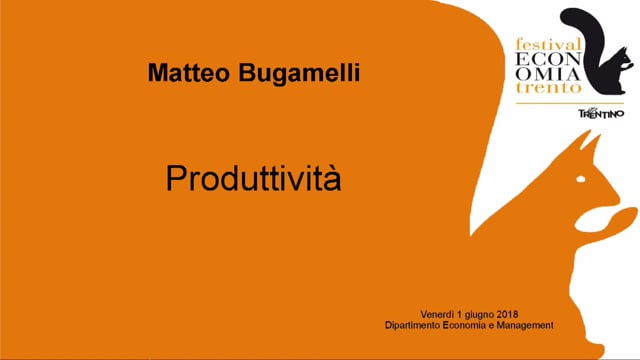
Produttività
Edizione 2018
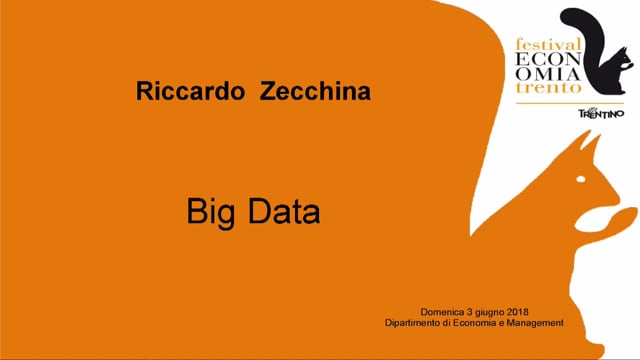
Big data
Edizione 2018
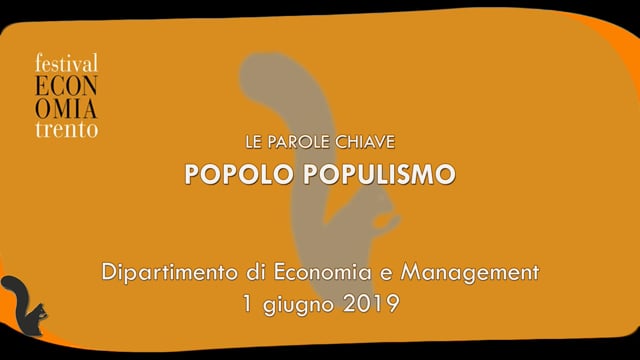
Popolo/Populismo
Edizione 2019
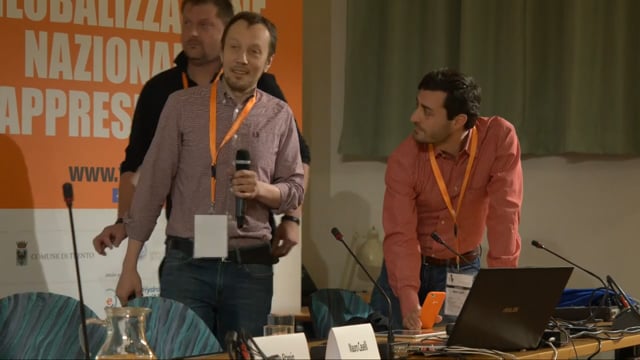
Globalizzazione
Edizione 2019