Polarization, AI and Polanyi's paradox
Incorpora video
Polarization, AI and Polanyi's paradox
I offer a conceptual and empirical overview of the evolving relationship between computer capability and human skill demands. I begin by sketching the historical thinking about machine displacement of human labor, and then consider the contemporary incarnation of this displacement—labor market polarization, meaning the simultaneous growth of high-education, high-wage and low-education, low-wages jobs—a manifestation of "Polanyi’s Paradox." I discuss both the explanatory power of the polarization phenomenon and some key puzzles that confront it. I finally reflect on how recent advances in artificial intelligence and robotics should shape our thinking about the likely trajectory of occupational change and employment growth.
good evening good evening a welcome by Massimo Russa and I'm pleased to introduce David outer the title of this meeting is the computers of people Palani paradox said that we are going to learn about immediately first and foremost I have to say that I feel like traveling on a time machine so in a few minutes I will be shifting from the past to the future and being here today after listening to what mr. cotterell's said mr. Kelly you know was the spending review commissioner have you told her so that it was impossible to replace even the let's say lowest performing workers in the tire ministries I'm talking about people carrying folders from a one area to the other of the entire ministries that despite the existence of email anyway it is however clear that forces are already working leading to the automation of tasks so that human beings have carried out the so far and this is what we are going to discuss tonight with our author the question that we often ask ourselves is where the machines are going to replace a human being certainly not but they will change so all humans Kizer whereas he believes there some tacit to skills that cannot be and therefore are not easy to be replaced by machines and this is what Palani used to maintain with its perilous paradox whereby we know more than we actually know and to understand what mr. otter is going to tell us at best I think we need to clarify a number of definitions first the definition of artificial intelligence tonight we're not talking about the Thinking Machines neither are we talking about our hope that is the movie that I believe in many of you have seen Nora we're talking about machines that can pass the Turing test that is to say a test that that would prevent any other party to know whether he or she is talking to a machine or another human beings but we're talking about machines that that rely on neural networks are similar to the neural networks of our brains are able to learn in other words that they are able to carry out a slightly more sophisticated task so then in they used to that is to say the cognizing a face or recognizing a character I mean what kind of work the brain has to carry out to enable human beings to recognize a cat and how can we make a distinction between a pornographic image and no pornographic one and there is a ruling of the sixties in Great Britain whereby the judge said that pornography something I can define when I see it but I'm unable to define it with a theoretical statement him so even though we are far from same machines that are able to fulfill any human tasks as forecast in the sixties the machines that do however play a key role in our daily lives a few months ago a company in Hong Kong and Hong Kong a financial company adopted an algorithm within the board of directors of the Kenner cast about in other words of the algorithm will be used to make financial decisions for the organization in the latest issue of a wired the publication I work for we published two articles the first was written by Arabella and the second by a human being and it's almost impossible it's absolutely impossible to make a distinction between them there is a research study by the Oxford the University by Michael Osmond whereby roughly 47% of our profession occupations over the next thirty years there will be automated or will be likely to be automated and this means that they can be carried out by computers and it's interesting to know what a David autor thinks about it so I believe you have a whole all heard about driverless cars or companies like a Boston Dynamics or minds that have been working on artificial intelligence and more specifically on deep learning but it's not just a matter of artificial intelligence because there are many other forces at work that are molding our present more than our future we're talking about a big data Moore's law permanent connection 3d printing and so on and so forth there are two authors I believe will be mentioned by Debbie tauter they are also MIT professors who maintain that these forces that are currently at work are leading to the to a new age at the Second Age or machines as they call it machines they are not going to replace all the human talks tasks so but they will be able to carry out - besides the manual tasks also intellect or tasks a low-level Winterthur tasks for instance a comparison of legal agreements or low level diagnose diagnostics in hospitals or financial investments and of course writing as far as I know and I would like to hear the opinion or David order on this a - I don't think there is a real contrast or between these as two schools of thought but rather a different opinion about the speed rate which of this evolution will take place and and the questions that we are going to answer tonight is whether we are at flex point similar to what we experienced in 1775 with the beta with the heat and with the steam engine and will the chain value be mustered by machines going forward what will be the jobs of the future and how education has to change it to keep abreast with this change in the uncertainty I believe it's advisable to get ready from an economic and also I think both of you as many scholars men dealing with it artificial intelligence are maintained so I'd like to leave the floor to David also now who is a professor the MIT and holds a number of offices in a prominent academic institution it was a director of the journal Caracas effectives he studied psychology and then had a PhD in a public policies there and before becoming a professor he was a teacher to economically disadvantaged disadvantaged children and adults in the San Francisco area and in Africa thank you very much thank you the title of my talk is why hasn't automation already wiped out employment or another way of saying it is why are there still so many jobs we live in a period of automation anxiety of people very worried regularly about technological change here are two recent examples so the book as was mentioned by Massimo by my colleagues Erik Brynjolfsson and Andy McAfee Race Against the Machine and suggestion of that book is that people are losing the race or just recently published rise of the robots technology and the threat of a jobless future and you can see why people are worried here's for example the rethink robotics this is Baxter this is actually developed by a colleague of mine at MIT Rodney Brooks and for those who were paying close attention it's uh you can order one for $25,000 it's supposed to replace a minimum wage worker now at that price there's no extended warranty so just to be careful be aware of that before you order um so what I want to say what I want to talk about today four points one why people are worried what are the proximate sources of their worry what gets people concerned - I want to put this in a little bit of historical context and I talk about you know previous episodes of anxiety about automation of fear of automation then I want to talk specifically about three technological revolutions one being the Agricultural Revolution one being the Industrial Revolution and the third being the current one that we're in which is the computer revolution it is it is revolutionary no question and what that means for human skills for the type of activities that people need to do then the last part I'm going to actually talk about the future and the challenge faced by artificial intelligence and robotics and also some of the progress now obviously I'm not a robot assist or an artificial intelligence researcher and I'm not a robot but I have I think I have a reasonably good sense I spend a lot of time talking with my MIT colleagues about this of sort of where we are and where we're going people have very different views so to make it clear I'm gonna give you one version then I'll draw some conclusions and at the end I'm going to talk by the way about jobs that will be here in 50 years jobs that you recognize now that will still exist 50 years from now okay so why are people worried well here's one thing that gets people worried this is just a picture of the rise of inequality this is in the United States this shows you indexed real wages from 1963 to the present by five levels education high school dropout high school grad some college college degree only graduate degree like a doctor lawyer etc PhD and you can see this fanning out this is for men this is for women so for example in the u.s. the real earnings of men with more than a college degree increased by 90 percent in this period and those with less than a college degree fell by about 10% if you look at women you see a similar picture in general it's more positive the slope was more positive but there's this incredible divergence so clearly what that's telling us is the demand for skills has changed we have more educated workers than we used to be and yet their wages keep rising ok that's one thing that has people weren't worried a second thing of course is a manifestation of that is declining youth employment or that's one man possible manifestation right so we see among low-skilled and be among entrants so it varies greatly across countries in Italy of course it's 40% you already know that in Canada and you it's 15 in Germany its 8% the fact that that vary so much across country should suggest that it's not it's not a universal phenomenon it's not one single cause so the combination of rising inequality and falling employment rates among some groups among young among the less educated and this is especially a phenomenon of the last 15 years has people asking is this the time when technology is making human labor redundant so just to give this a little bit of history here's an earlier era of anxiety about automation it's occurred during the Industrial Revolution Ned Ludd was actually not necessarily a historical figure but the people took his his his the stories inspiration and they went around smashing power frames which were an early water-powered Louvre English lute weaving machine and this happened sporadically throughout Industrial Revolution and what these people were responding to understandably was the sense that they had a set of valuable skills they were skilled weavers and artisans and they're being replaced by machines operated by children right most of the work in in textile factories was done by children who were who lived and work in the factory they had low skills their main skill was they were dexterous they could work quickly with their hands and if you weren't quick your fingers would be taken off by the machine it was quite dangerous um and of course they they were right to be worried because it did devalue their skills it created lots of other wealth right the Luddites were not people protesting against lower prices of clothing they weren't protesting against the fact that they could they could actually you know or own more than one shirt and pair of pants but it was it was directly disruptive for that group of workers more recently another era of automation anxiety so as many of you know that you know that what brought the u.s. out of the Great Depression was the Second World War when all the machinery of production was turned towards the wartime effort and there was a concern after the war ended that we would return to the Depression era because there wouldn't be enough demand there wouldn't be enough work and what made that problems especially salient especially on people's mind was that productivity was rising extremely fast it was rising at the rate about three to three and a half percentage points per year so for example in 1964 President Johnson set up the blue ribbon National Commission on technology automation and economic progress and they were there to deal with a productivity problem and the problem was that we were getting too productive and similarly in 1974 the US Department of the Interior wrote that leisure thought by many to be the epitome of paradise may well become the most perplexing problem of the future now you may ask yourself why does the US Department of the Interior have this worry well the US Department of Interior runs the u.s. national parks so they were concerned that all these people with all this leisure would descend on the national parks and they would be overwhelmed by demand and this by the way was emblematic this was the Machine of this era of the mill Milwaukee Matic industrial machining tool and it was a computer numerically controlled machine and you can see it's quite an advanced device because the man operating it is wearing a lab coat so what actually occurred in this period of incredibly rapid technological progress well a number of things occurred one is that over the course so this is as long as they have consistent data in the um giving us data from 1890 to 2014 in general total employment rose throughout this period right male women's employment went from unpaid employment in the home to paid employment in the workforce such that about 60 percent of women working in the u.s. actually relatively low by world standards and male employment it reached a peak in 1950 it's been coming down but most of that's not a bad thing right that means in in general we actually have enough resources for not everyone to be working all the time but in net the employment population rate has risen throughout a century despite all this automation similarly if we look at incomes so in general you would say there's a kind of a fivefold rise of real incomes in this period measure in terms of goods and services that people can consume and another way to see that here's a much more dramatic way to see that it shows you the fraction of income spent on necessities on food clothing and housing the three things that everybody needs and that has fallen from 85 percent to about 55 percent leaving essentially 45 percent for necessities necessity I'm sorry to me for for discretionary purchases right those things could be leisure they could be medicine they could be transportation but that's an amazing transformation of the sort of of real material wealth and that of course ignores all the improvements in health in longevity in safety in quality of food quality of experience so this the the record so far has been incredibly positive right despite you know one or two hundred years of people worrying about automation reducing employment it's had the effect of dramatically increasing living standards without having any net reduction in employment now let's talk about the future so the fact that that has been the case before everybody will knows that and if you say to people who are making this argument that we should be concerned about automation they will say this time is different now it is different it's not the same so it's reasonable to ask the fact that it's never that has never been a that technology has not created long-term unemployment in the past doesn't mean it cannot right it'll be too simplistic to say it's never happened before it cannot happen again so let's talk more specifically about the nature of technological change that we're experiencing so over the course of the last hundred and seventy years we've undergone at least two prior technological revolutions plus the one that we're in the first one was the Green Revolution right the invention of fertilizer of irrigation of genetic manipulation of seeds reduced agricultural employment in 1840 it was 70 percent of employment in 1900 was 40 percent in 1950 was 11 percent in the u.s. at present it's 2 percent of employment right and it's not 2 percent of employment because we stopped consuming food look around America not stop consuming food I it's not because we're importing food it's because we become so productive right now that's a massive benefit but it's caused a huge movement of labor out of Agriculture where do people move well initially they moved into industry the height of employment in US manufacturing was around 1943 at which point there were a little bit over 40% of US workers were manufacturing at the same time there was probably another 20% in agriculture and the rest were in services and then as we automate an advanced as well as international integration of markets people moved out of industry again and into services services is a huge category right it's everything from software to hospitals it means everything that basically isn't agriculture and manufacturing and primary resources like metals and mining and so on now let me say these two transformations were very disruptive most people would say they both were hugely beneficial they didn't happen automatically so one could look at this and say see it always works out part of the reason it worked out is because people were forward-looking so around 1918 ninety people in US farm States realized that their children were not going to be employed in agriculture they saw that Pro he was increasing fast enough there was not going to be that much farm employment so what did they do they started what was called the high school movement the idea of the high school movement was to send every US born child all the way up through high school that was considered a radical idea at its time it was radical for two reasons one it seemed frivolous seemed excessive why does everyone need an elite education why does everyone need literacy and numeracy writing seems too much it's expensive all those years they could be working on the farm they're in school the other concern was maybe most people aren't really smart enough to do that right you know you're asking people to you know it's like I suggest I said everyone in this room should go out and get a PhD maybe you'd say not everyone's really prepared for that well that was like sending everyone to high school at that point right that investment in human capital was one of the most central decisions of the US history in the 20th century that is probably you know along with along with you know other good force from the United States that had a a great deal to do with u.s. success and growth because it made people adaptable flexible it made them innovative allowed them to adopt new technologies successfully and become technological leaders now we're obviously in another period we're in a period of computerization it's not the same as the Green Revolution clearly it's also not the same as the simple Industrial Revolution what's different what is different this time well to put that very concretely I'm gonna give you a very specific example this is what many people think of as the first commercial computer this is the jacquard loom front this is an example from 1801 and is an automated loom that is controlled by punch cards and what makes it different from all the commercial machinery that preceded it is that it's a symbolic processor meaning it acts on stored representations of information right so these cards this is not a picture of what it's making its instructions it's telling it to lift arms right to use different threads there is an example of surviving program made by jacquard himself with 10,000 cards that weaves a silk portrait of jacquard you knew what he was doing I this so this is the first commercial symbolic processor this idea of symbolic processing was so powerful in fact did your car bloom was the inspiration for Herman Hollerith he's the person who invented the paper punched card it was used to read the 1890 u.s. census the first machine read census of populations he joined with Thomas Watson to found IBM so this idea was very foundational I would argue that modern computers are very much like the jacquard loom they follow stored instructions they carry out those instructions precisely rapidly inexpensively they don't think for themselves right they don't make judgments they follow instructions that describes 95% of what computers do doesn't describe the other 5% so what has changed in the Queen D cake was a chimera and they posed to me the real cost of carrying out a million calculations from 1850 to 2010 so this is a logarithmic scale so each drop of this size is a hundredfold decline for a hundred ten thousand ten million so on hundred billion excuse me so from 1850 to 1950 there basically is no progress why is there no progress well people are there's more literate people around but they're still expensive so it doesn't actually get cheaper to do a million calculations then around 1950 we enter the vacuum tube era of computing right during the Second World War what Alfred turbine was working with for example and and then we enter the transistor area which is basically a very small inexpensive way of making a vacuum tube a vacuum tube is just a switch then we move into the integrated circuit era the microprocessor era and in between 1950 and 2010 the the cost of carrying out a million standardized instructions Falls one to two trillion fold right it goes from expensive to essentially free cost of computing for most activities is almost zero not zero that's a big change right and that really creates a strong economic incentive to figure out what we can apply that to because anything we can do with computers we can now do one or two trillion fold more cheaply than we could do 50 years ago so it creates an enormous incentive to take this technology and figure out what can we use this for because it's now it's like discovering you know endless electricity or an infinite supply of oil right whatever you could do with oil you can now do for next to nothing that's what computing power became like what do we do with that well the first thing we do with it is we substitute things that look like what the Loom did things that follow well specified procedures codify Abul instructions right there are some jobs that really look like that bookkeepers assembly line workers people who follow procedures well scripted procedures those tasks can be automated readily you think about what bookkeepers do if you think about what accountants do what people who type and file and calculate people who look up information right people who sort through and retrieve people who duplicate these tasks are readily automated and have been automated we see a lot of that however there's at least two broad sets of activities that we are not very effective at automating one is what you might call abstract tasks these are things that involve creativity problem-solving intuition communication with other people empathy leadership management these are things that require a kind of mental flexibility that is not easy to codify these are found in the in professional and technical managerial jobs those activities have not been automated by and large in fact arguably they've been complemented by the technology right you can be a more effective researcher if you have access to lots of data you can be a better lawyer if you can look through all the cases right you can be a better architect if you can calculate and redraw a building as quickly redraw the engineering plans so there's complementarity there the other set of tasks and many people find this surprising once you think about it it's obvious there are lots of dexterous flexible physical tasks that we do that we're also not good at automated right so you know working as a flight attendant or a personal aide or a waiter or a cleaner or a security guard I'm even going to say truck drivers for a moment I know you'll disagree um these are all tasks that have proved extremely hard to automate and I'll talk about why in just one second but there are many more of these if we look around the industrial world there are fewer people working as secretaries there are fewer people working as production workers there are more people working as lawyers attorneys scientists there are more people working in personal services working restaurants working in cleaning working in home health in assisting people you can see this in a variety of ways so these are data from the US and these line up occupations according from lowest paid to highest paid in broad groups and these bars correspond to percentage changes in employment over the period of the 1980s the 1990s the 2000s prior to the recession 2000s after recession what you see on the left side you see personal care food and cleaning protective service these are these manual jobs many of them are mundane and the sense that they're they're not intellectually demanding but they require a lot of flexibility those have been growing as a share of employment if you look in the middle particularly production workers office administrative workers and sales workers those have been in decline many of those jobs are very codifying we see a lot of direct machine human substitution now you look on the right side managers professional and technical workers these are jobs that are abstract task intensive right they require that creativity problem-solving that have proved very difficult to automate so far you can see a similar pattern across the EU so this presents this is from paper by goose Monti and Solomons they look at 16 European Union countries from 1993 to 2010 and jobs are categorized into low-paying that's the blue bar middle paying that's the red bar and high paying these are the green bars these roughly correspond to personal services technical and clerical workers and the high paying or professional technical managerial what you see is this pattern of declines in the middle skill group across all these countries this is a broadly shared international phenomenon and the important part of this not all this has to do with computerization with basically replacing those codify Abal tasks so why haven't we replaced the rest right why are people still doing these jobs and why are they still doing these jobs why don't we just computerized them all so this brings us to polanyi's paradox Lonnie is a philosopher and a scientist and he wrote a book called the tacit dimension and you know just one sentence I like to quote we know more than we can tell what he what that means is there are many things that we do regularly almost effortlessly that we don't explicitly know how to do that we don't know the procedure for doing them the reason why is that true if you think about these kata file tasks clerical work mechanical work you know doing repetitive assembly work these use formal tools that we developed for that purpose counting mathematics logic right these are all things that we taught ourselves so we can teach machines because we produce the rules to do them if you think about these non routine capabilities spoken language sensory motor skills physical effects flexibility judgment intuition creativity these are things we didn't create those we evolved those right the things that we developed not consciously or intentionally they developed them as we evolved so to try to teach a computer to them we have to figure out how we do them we have to reverse-engineer those activities right the reason they're so challenging is I can teach a person how to do algebra but I can't teach a person how to walk right I don't know the procedure for walking I just do it right so it's easier for me to teach a machine to do algebra than to teach a machine to walk walking is a problem I don't understand I just do it I know it but I can't tell it and that has been a huge challenge for automation is we don't understand many of the things that are easy for us it's easy for someone to vacuum a house or to empty a dishwasher or to you know look around a room and recognize all the people even if you haven't seen them in their their you know hair color has changed gray whatever on it's very hard to have a machine do those similar tasks that's a huge eyes remained a huge challenge now there are two implications of Paul II's paradox one is kind of an engineering implication and the other is an economic implication an engineering level very straightforward we can't automate what we don't understand by and large there's exceptions to that but by and large we can't automate what we don't understand so it means lots of things that don't get automated stick around whether those are managerial tasks or whether those are personal services tasks an economic implication is that tasks that are not substituted by machines are generally complemented in other words made more valuable and the reason for that is that most jobs involve a variety of things that have to be accomplished together right so if I'm if I you know I'll give you a couple examples if I'm a doctor I both need to run tests and do diagnosis and then I need to come to a judgement and prescribe a medication well if I can run those tests more quickly and access better information that means the value of the rest of what I do is increased right because I can help more people or do a better job right so the fact that part of my work is replaced means I become more valuable not less valuable similarly if I'm a if I'm a person working in a restaurant and I have a bunch of machinery so instead of serving 10 customers in a day I can serve 50 I can make I can cook faster I can make more lattes though not fully automatic just a little bit of help then then my time is more valuable right if I'm a construction worker right and I can use powered equipment to dig instead of using a shovel then that increases the value of my intelligence right of making the right decision of deploying the machinery in the right place so in all these ways right technology it's not simply that the work goes away and nothing's left it means the work that remains becomes more valuable as a consequence of increasing productivity in what is done by machinery now so here let me give you just kind of one surprising example many of you are aware of course of in the last 30 years automated teller machines those Bank machines are everywhere nowadays you would think right so this this refers to ATMs installed so between in the u.s. between 1970 and 2010 the number of teller machines went from zero to 400,000 well in the same period the number of bank tellers went from 300,000 to 500,000 now that's actually a decline as a share of all employment but it's still a big number right what are all those bank tellers doing well once banks had ATMs they said gee we can put ATMs in more places and we can open little branches well if you open a bank branch you might want a teller there to help solve problems not the problems in ATM but let's say someone wants to make a deposit or let's say they have overdrawn cheque or they want to open a new type of account right it turned out that the decline in the in the cost and providing these basic services created more demand for people to do the complementary services and that's not always true it's not not the case in every innovation increases labor demand directly in that activity but even in something as where you think it must be the case that computerization has eliminated all the people it turns out not to be the case let me give a broader example there really three factors when you think about how technological change affects what the value of Labor of what people do that's remaining that's not only made there kind of three factors that are important one is workers benefit if their job tasks are partially but not fully substituted right so if I'm a if I'm an engineer and I do some hand drafting and then I get access to CAD software right I become my expertise in drafting becomes more important not less important or if I'm a writer and I get a word processor so I can edit drafts more quickly right that makes me more riah but on the other hand if I'm a person who sets type at a newspaper and all of a sudden there's a computer that does that entire job it obviously is not good for me right so it matters whether you're fully substituted or only partly substitute that's one factor the second of course is how elastic demand is for that service so in general automation reduces the price of making stuff so as it reduces the price back in either increase or decrease total demand for labor in that activity depending on whether demand is elastic right so in agriculture after productivity rose enough turned out there was a finite amount that people wanted to eat and so eventually employment contracted because demand didn't grow fast enough with declining price to create ongoing growth and employment however what we see in medicine is just the opposite as medicine has improved and the ability of doctors nurses and all those professionals to improve health right demand has grown more and more right a contemporary doctor could do all the good a doctor could do 100 years ago in ten minutes mostly because doctors couldn't do much good a hundred years ago i but i but as we improve the product right demand has kept pace more than kept pace a third factor and this is a important one in the subtle one is the elasticity of labour supply in other words the number of people who can do the job so for example i said that a lot of these personal services like cleaning like food service like child care they're not becoming automated at a rapid rate does that mean they'll start to pay a lot more not necessarily and the reason is because there's a huge supply of people who can do that work right even though they're hard to automate they're relatively easy for people they don't require extensive schooling they don't require extensive training so even as they grow as a share of employment that doesn't mean that wages rise in all those activities and i think a lot of the concern that people have about the changing characters of jobs is those jobs that are growing at the bottom those personal services are numerous but they're not highly paid okay so I won't go into that one so now I've given you a kind of an overview of I think you know what is been happening but let's talk about what's going to happen right so you know I've sort of said computers can't do this computers can't do that and you're sitting here thinking they will that's gonna happen very soon this is irrelevant and we can come up with examples all the amazing things they have done driving cars reading legal documents interpreting x-rays aren't computers basically going to do everything very shortly so or is polanyi's paradox that we don't know how to do what we do is that now irrelevant because we'll figure it out or computers will figure it out for us well there are two different angles of attack two different approaches that engineering and computer science are taking to overcome polanyi's paradox the first one is to basically change the world to make it machine compatible explain that one second the other is actually to teach machines to learn so let me talk briefly about both of those okay so environmental control environmental control means making the world simpler for computers right if we can't make machines adaptable let's make the world simple and we do this all the time right just to give you an example that's not from the computer era right if you you're the car that you own or rent is safe it's efficient it's fast it's reliable it's also incompetent on most of the world's surfaces you need a almost perfectly smooth and flat surface to drive on very little of that surface exists in the world so to make the world car compatible we pave it right so in the United States the area the paved area of the United States is equivalent to the size of the state of ohayo not a small state why did we do that to make the united states car compatible right we simplified the environment to make it compatible with machines that's a one approach to automating more things so this right here is the Kibo robotics warehousing system Kiva is a MIT based startup is now owned by Amazon these little pods here are the robots okay they look like little vacuum cleaners all they do is they drive under these shelves and pick them up and they drive the shelf somewhere else they put it down again how does this how does this automate a warehouse well what happens is as stuff comes in to an Amazon warehouse the they enter into the computer what has arrived the robots pick up the shelves and they drive them to where the the people are at the loading docks the people put the objects on the shelves and they tell the computer what object ISM on what shelf right that the wrote the shelves can't see the objects they can't feel the objects but they're told that those objects are there then the shelves drive their way back into the warehouse right on this flat surface they pack in real tight because no one needs to get in there between them and the software organizes the warehouse right so if people routinely they order if they go to Amazon and they order a book and a movie and a case of beer then the book movie in case of beer shelves will all be next to one another right that's what the robot the software will do then an order comes in what happens well the robots the order goes out the robots drive around to the shelves they pick up the shells that have the objects that were ordered and they bring to them to Tim the human pick worker what is Tim do well he stands there the robots drive up to him with the objects on the shelves a laser pointer points from the ceiling to the object Tim picks it up puts it in a box sticks on a label gives it to UPS right ok so what's happening here well basically what the machines are not good at is objects they're not good at recognizing objects they're not good at moving around on uneven surfaces they don't have arms or eyes or hands so what Kiva said is we'll just take almost all the job the routine part we'll make will simplify the organization and then we'll just use human hands and eyes for the last bit putting stuff on and taking them off right and that's one example of changing the environment to make it machine compatible I'll give you another one the Google car the Google car you may think well the Google car drives on roads like you and I and that's true but the people computer scientist will often say the Google car drives on maps not on roads what that means is for the Google car to go drive in some place you have to have a bunch of of Google engineers who first go and map it not just like the map you see on computer but down to the inch here's where the track here's where the traffic lights are here's where the curbs are here's where the stop signs are and then when the car drives in that area its scans the environment to see if it matches the map if it matches the map it says okay I can drive here now I got to look for hazards right other vehicles people in the street people who might come on to the street and so the Google car drives on this map and it compares what it sees to what's on the map to make sure that that's it's where it belongs and it just needs to make these adjustments to small changes but if you were to plunk the Google car down in the middle of a place where it did not have a map it couldn't drive and in fact when the Google car encounters something that it is not expecting it hands off to the driver this is actually a huge problem in automation in general the so-called handoff problem right this is this is what for example brought down that Air France jet some years ago basically the computer got overwhelmed hand off to hand it off to the operators they didn't know what was going on either it's hard to get them to keep paying ten I mean my point being that that's one example at the Google car is another example of changing the environment in some sense to make it machine compatible and we will see a lot more of that right so when when robots come and operate our appliances right when they drive our vehicles when they go up and down stairs the environment will be changed to make it easier for them they won't have to use their eyes and their smell the way we do they'll be radio tagged right so eventually all roads will have radio tags that tell the computer where it is doesn't have to look for traffic signals right it will read a different type of data okay there's a second approach so one approach to overcoming polanyi's paradox is changing the environment to make it machine compatible another approach is to try to get machines to infer the rules so if we don't know the rules maybe we can have a computer look at this look at the world and try to figure out what the rules are so for example one idea of this is to show a computer a large database let's say we show a large database of pictures and we tag them say this is a child this is a chair this is a car right this is a latte and then we don't explain what those things are right we just give it those tags and then the computer uses a statistical process called statistical machine learning neural networks deep networks but basically to to maximize the probability of getting a correct yes right so we show it a billion objects with labels then we show it a billion unlabeled objects and ask it to guess right and by that process what's called training right we never tell the computer what the rules are right it just makes the jump from input to answer and tries to get a statistical Association of those things this process is all around us now so for example if you use if you talk to your phone to ask them to do stuff it takes your your speech and compares it to a big database of what other people have said right to try to guess what you mean or if you start typing at Google if you just start typing Google and you start typing you know ta Y lor write few letters in it'll say Taylor Swift right before you get partway through that right it's not because there's a Google programmer that has you know entered a line of code that says say Taylor Swift when you start typing it's because the machine has learned or has inferred that if someone starts typing that sequence of letters they will be typing Swift shortly thereafter right that's machine learning that's the idea is that the Machine infers from from behavior what to expect here's another example of that this is from Google Labs and this is a network of sixteen thousand processors that was shown a database of billions of images and not told what any of them were and it just tried to organize them into groups not with the name by just saying these things look like one another right so it came up with a group of things that all appeared to be cat faces right there were faces of cats well not all of them this one's actually two coffee cups but it looks kind of like a cat face right and what this example shows you actually the coffee cup is important it shows you both the power of machine learning right because the machine it was given no information it wasn't told what to look for and yet it organized things into groups that were like each other but of course it also shows you the limitation because it had no context no reasoning about what it was looking at right it was very it would be very difficult for it to infer that that's not a cat face right if you showed this to a child they would immediately know that why Vicki why does this not look like a cat it doesn't have eyes doesn't have yours right there are no eyes here right you know and also if you look closely the surface is incredibly smooth it doesn't look like an animal it looks like a machine made object right but of course for a computer to do that type of reasoning it would have to have a much richer model of the world so Carl Sagan the sort of popularizer of scientists said if you want to bake an apple pie from scratch you need to first invent the universe right so the difficulty here is trying to do a very narrow task but to do the broader task you need the whole universe of understanding let me give you a more recent example many of you have heard of Watson the IBM computer that won the game of Jeopardy in 2011 and it did win it was amazing it also got at least one question spectacularly wrong and it's interesting to see why it got it wrong so the question was US cities and says its largest airport was named for World War two hero its second largest was named for world war ii battle so the correct answer is Chicago right the main airport is O'Hare the second Airport is Midway I even said guessed Toronto which is a second largest airport is Waterloo not a World War II battle why did it do that well if you were to type US cities World War 2 battle second largest airport into Google you would get a list and depending on how you typed it you might very well get Toronto right because Google doesn't understand that US cities is non-negotiable right that it had to include that it just does a statistical pattern match it's not reasoning about the full answer right so that's why this you know impressive as it is you can see why it would make such a stupid mistake right and it's because it's not reasoning in the same way you would that doesn't mean it's not useful it doesn't powerful but it's different you final example what is it chair so there's a long that there's a long history of computers trying to categorize objects by looking at them and it's difficult because objects come in many shapes and sizes so if you said a chair needs to have four legs well you would rule out many chairs that have three legs if you said a chair needs to have a back well you would rule out a stool if you said a chair needs to have legs and a bottom but doesn't have to have a back well then you would include a table right so it would be hard to tell based on describing characteristics so when people look at objects and ask what are they for they don't just look at their characteristics they think about how would they could they be used right so you can look at these three objects they all look somewhat chair lighting right you could say that looks like a chair looks like a chair you think Oh would I want to sit on that no uh and so when you answer that question about recognizing object you're using a lot more reasoning than as typical artificial intelligence device would use and so the point I'm making is there's a huge amount of knowledge and expertise and understanding that goes into very basic human activities and that's a huge challenge for automating them it doesn't mean progress is not being made but the idea that computer successfully categorizes some objects or translate some text that's not intelligence the way that you would apply it doesn't mean it's not an important achievement but it's a long way from what you can do okay so let me say let me I'm gonna come to an end now in just a sec let me say first of all one could be too pessimistic about how progress so I don't want to suggest progress is not occurring here's an article from Popular Mechanics as written right after the Second World War talking about the ENIAC which was a computer used in the United States for the war effort and they they made the bold prediction where a calculator on the Eenie AK has 18,000 vacuum tubes and weighs 30 tons so maybe 20 thousand kilos the computer of the future may have only a thousand vacuum tubes and way perhaps you know 1000 kilos right so they were way too pessimistic about how fast things would change things changed a lot so the progress is remarkable I don't mean to suggest it's not okay let me come to some conclusions um so let me say here I'm gonna go out on a limb I'm gonna tell you jobs that will exist 50 years from now okay I want to be very specific so that I can be proved wrong okay building and installation workers plumbers electricians contractors installers technical and creative experts and leaders architects doctors professors what lawmakers develop people who develop and test new ideas like scientists researchers engineers people who fix things you move fix cars planes masonry people who fix robots people who fix robots that fix other robots um teachers lots of teachers there will be lots of teachers entertainers athletes musicians actors chefs comedians personal helpers of all kinds people who help with careers who help with physical health with mental health with diet and beauty and parenting sex workers sorry there will be sex workers 50 years from now there'll be lots of robot sex but it won't really count okay so to conclude one people recognize the way machines displace people but they don't tend to recognize the way they compliment that's important to recognize that complementarity - it's very hard to anticipate where the new jobs will come from so if you'd asked a farmer a hundred years ago look I'm telling you farm employment is going from 40 percent to 2 percent what do you think all the farmers are gonna do they would not have said hmm software healthcare business services tourism right it's very hard to figure out and so when people tell you it's not gonna happen this time I say how can they be so sure that they know what isn't going to happen right why are they so confident that their lack of imagination is proof that the world won't advance that people won't come up with new ideas that's actually a very bold prediction to say that we won't come up with new activities for people to do I don't think that history favors that prediction third a question and I think the anxiety that a lot of people feel it's not really about the number of jobs it's about the quality of jobs right the problem that people are concerned about it I understandably and myself as well is that a lot of the jobs are sticking around are not going to be high paying jobs because lots of people can do them right and the challenge and the response to that and the response that we've had for a long time is education right is raising the quality and quantity of public education out keep people relevant if we took the workforce of the 19th century and plopped them put them in the present most of them would not be employable because they would be illiterate in a numerator what made them employable is that we educated them and that's an ongoing challenge and it's a challenge we face now and arguably we're not advancing as fast as we need to on that front finally what if I'm wrong what if the robots take all of our jobs well that's good news right means that we're very wealthy means we can have the standard of living we have now without actually working it creates a problem but that problem is an income distribution problem right that's problem of how do we share all that wealth the way we distribute wealth right now is based on labor scarcity you have labor people want to pay you for it that's good that keeps you employed for a lifetime if really all labor were abundant if there was too much labor because machines provided all we may have vast wealth but we would have to use a different system of income distribution to share that wealth that's a big problem I hope we face that problem I don't think we'll face that problem let me finally end so Herbert Simon the only Nobel winning economist who was also a computer scientist was also worried about this issue 50 years ago and he wrote the world's problems in this generation and the next are problems of scarcity not of intolerable abundance and when people are worried about automation and a technological advance they are worried about abundance at some level right and I don't think we've ever faced that problem the problem of having too much too much productivity too much ability to create too much stuff we don't I don't think and I think Simon was right 50 years ago and I think he's correct now although he's long ago passed so maybe he would have changed his mind by now but the the issue that we're facing we face issues of income distribution we face issues of poverty throughout much of the world the issue that we have of having too much prototipo of being too good at stuff of making too much too quickly too readily I don't think that's a major problem that we will face in the very near term that's it gracias order photo thank you very much a professor author we have a few minutes for the QA session please use the microphone and so what what are traduced driving that so he isn't the case that essentially managers produce something that is not measurable and so it's difficult to actually compare if ever you switch it you can take the machine and I produced thousand which I simply machine produces 10,000 which estimation is better so with managers you can do that and so it's not the case that essentially society has responded to this technological progress and the middle being squeezed out by moving these people into manager their jobs so I think managerial like many jobs that stick around they have a variety of tasks some of which have been automated so to the degree managers were just information movers right coordinating who did what today there are many fewer of them but in general managers also perform kind of supervised supervision valuation motivation they deal with people's feelings right so all of those things are reasons why managers add value right there are still secretaries and Clannad clerical workers in the world but they do something very different from just typing and filing and answering phones right they solve problems they deal with complex situations that they don't have a computer program to do for them so almost all jobs that exist have been transformed in the sense that the repetitive or just the information processing component has been moved into machinery what managers do what secretaries do what drivers do what factory workers do the things that they do now are things that require something else some additional problem-solving some additional discretion some additional flexibility if it's just about moving information around it is almost sure to be automated hi thanks for your speech was very very interesting my question is what do you think that government should do in this world I mean they they have just to provide a better education everybody should have a PhD or they have to resist the automation process or they just have to put public money to protect the weaker workers like blue-collar workers or maybe they should use money to finance automation because in the short run the outcome will be better if the process get faster and not slower as politicians you usually try to do Thanks good I mean my answer to all - almost everything you said is yes except the last one so yes governments need to invest in education and providing high quality universal education is one of the most important things that good governments have done over the last hundred years it's also the case now the government's role is to protect people right there's there's always going to be people who don't make it to that level and those people are going to need assistance and there's a these are complements right on one hand investing in educating people on the other hand protecting and assisting people who are not successful and of course we're getting wealthier so we can afford to do that and then it's also important to recognize that not everyone has to have high education for people to benefit from high education so if you make if you increase the fraction of the population with a college degree from 25 percent to 50 percent well that benefits the people who don't have a college degree they're now scarce er right when someone needs to hire someone for cleaning or manual labour or in-person services or vehicle driving or for heavy physically demanding job there are fewer people to do that so raising the education doesn't just benefit the educated it also benefits people who have less educate because it decreases their supply and therefore increases their value so there's no one solution to this but the one thing it has has no successful history is resisting the texting technology of sort of saying well let's not allow that to occur it's going to occur if not here will occur somewhere else you want to take advantage of it while simultaneously assisting people to be successful to work well in that environment I think there are two more questions so I got a question about the technical progress in your presentation very interesting I must say you started from the assumption of that technical progress is something exogenous and that machines can either complement or replace a human activity but is the technology progress that can be conceived in a different way so instead of considering it as an exogenous factor could be something that is more endogenous and therefore can again replace more they supplement or complement the certain activities that the technology is not completely exhausted that its shaped by market demand you can see this in many ways one specific domain where you see it is in medicine right in pharmaceuticals right pharmaceuticals are developed to treat cancer but not to treat malaria why because people in the rich world have money and they face cancer risk but they don't face malarial risk so clearly economic incentives drive the direction of innovation in a way that's good because in general if it means something becomes expensive there is an incentive to automate it so you know you should really be trying to automate high skill labor right people PhD they get paid too much and you should be trying to figure out how to use less educated workers because they're inexpensive at present right so in general the automation does go in the direction of trying to reduce the cost of things that are expensive things that are scarce and trying to make use of things that are abundant I don't know how we shape the progress of workplace technology to make it go more in that direction I think it's a great question and I don't and I don't think it's impossible that could be done but I also don't know how to do it and the market forces that do Drive automation are so powerful there's so much wealth money associated with them that it's hard for governments to shape them I don't know okay hi really thank you for the for his pitches was really interesting I have just two easy questions first is you said before that the beer of the box of beer was next to the book that specific book okay so it's because someone implemented their choice before okay and my question is would be in the future we'll be robots in the future able to decide to put the book that book next to the box at the beer box the answer is yes of course but because someone implemented it okay again so in the future we'll be robots or computers be able to identify space of improvements find a solution and implement the solution by themselves okay without implementing anything and besides I wanted to ask if in the future what we call in Italy humanistic knowledge so like philosophy logic and this this universities as well will be more important than other universities like engineering or something like that thank you very much let's see so uh good questions on the first question I will say that there there's as far as I know there's no current artificial intelligence that is able to sort of think of a solute a new idea or you know look at a you know produce a different way of doing things or have a creative insight I don't want to say that can't happen but it's very far away the people who work on artificial intelligence sort of consider that a problem that's you know way up the food chain from the problems they're working on most artificial intelligence problems are very concrete they're how do we recognize the people in a room how do we tell the cars apart from the buildings how do we take this chunk of text and translate it and yet keep its meaning right so the question you're asking I don't want to say it's impossible but I don't think there's even any real science on it at the moment on the second question of the humanities and engineering I think that those things are valuable now because they're valuable in even if you know whether or not you're interested in Renaissance art the ability to to articulate to express to debate the the ability to think clearly and analytically about any topic and communicated that is a scarce skill there you know at MIT I encounter students all the time who can't write and that's a huge problem for them a bit of a problem for me too and so those human skills that the sort of flexibility creativity the intuition the expression those will continue to be very valuable and many of them are learned in humanities they're more valuable still when they're applied to a specific domain that has expertise whether that domain is philosophy whether it's engineering whether it's medicine right whether it's entertainment so cultivating those specifically human skills and then applying them to the right domains is there will be there is and will continue to be tremendous labor value in doing those thank you I have a very specific question actually I'm working on income distribution and so I'm quite familiar with your paper on polarization and automation and actually if you look at things a bit more closely when you think about polarization and your slide clearly show that we're not really seeing an implication in terms of increasing inequality but rather polarization polarization means that the middle-skill sort of job go down but actually you have an increase in the top wages let's say or the middle higher middle wages but also in the low in the low wage so basically you could see an increase in accommodation and a reduction in equality and an increase in a polarization you know you have vast urban and we have sort of extremities you know polarization as opposed to inequality so the issue there is not really increasing inequality but rather the issue of the middle class like in the United States not really the same it has different implications also in terms of political economy and instability so what are your sort of predictions are you saying that eventually we're going towards more polarization eventually less inequality more instability smaller middle class or rather increasing inequality or we see in other words divergence as Wolfson authorized between polarization and inequality and the last question how do you associate that eventually with the sort of consensus or more or less consensus around the decline in the US labor share or more generally in labor share okay that's a lot of questions so the point that I was trying to make sort of informally during the talk is that polarization of employment doesn't necessarily lead to polarization of wages because when we have growth of high skilled professional technical and managerial jobs we just hinder eyes because the supply is quite inelastic right because it takes years to send people to med school or law school and so on when we talk about when you see growth of in-person services like food service like flight attendants so on the supply people who can do those things is quite large and so the only tech lease in the United States the only time we've really seen wages rise in those services just is when labor markets were very tight and you had to pay people to do them and so there's something else in the are the u.s. experience over the last 30 years has primarily been one of rising employment in low-skilled work without much an increase in low CEO wages and the explanation I would offer is that as people are displaced from the middle they tend to compete down right when they're displaced from clerical work from industrial work the jobs they can do our services much harder for them to go be an engineer be a lawyer so I'm not as optimistic as you about polarization actually leading to convergence at the bottom I see much more of it leading to a large number of not particularly high-paying jobs your second question was Rahmi I apologize labor share oh yeah yeah the labor share thing right so this is there's there's evidence that the share of of total national income paid to labor has declined over the last ten years in a number of countries the and and that's surprising because it's been relatively stable for the last 70 um I think it's too early to draw a conclusion about that I think that the evidence is relatively tentative this is an unusual period and I don't think we really know we don't know if that's going to continue and we also really don't know the cause there's claims that we do there's always it's always the case that people's claim understanding precedes certainty the claim is that this has to do with automation and falling price of equipment I think it's it's just as possible that actually has to do with concentration of industries and the growing concentration of many sectors and that concentrated industries have large firms that are very profitable and are very capital intensive and don't employ a lot of labor so I don't know I think it's something to watch it's not it's not something to just dismiss out of hand but I think it's it's too early to conclude that this is the we've now entered the time when machines just start replacing all the workers when a queer and from an economic history point of view you showed the Sarika evidence of technological revolutions and my question is whether the let's say the results would have been the same observing not the US labor market but the labor markets of the country where those products were sold actually were exported as an issue the mechanization of the cotton fields in u.s. at the impact on not only on US farmers but on Italian farmers that had no way to find a job in repairing those those machines let's say so the twenties why they're on global in a global globalized economy where those but just few workers can produce what all the workers needs and let's say if everyone starts working at those productivity rates there is no demand for buying all the goods produced in all the world we have a history of few countries and we are observing just the technological leaders labor markets effect so the question is whether when on a global scale if those technology will be available to everyone whether we have to like start questioning who owns the machines rather than which machines to invent next thank you so I think you make an interesting point and is the case that we could have a technological revolution in one country that would raise wealth but reduce demand for products from other countries right you can imagine if we really automated a lot of textile work the handwork it would be bad for Bangladesh in Pakistan for example so I think that's right it's not it can it doesn't not everybody wins right away at all and it can be extremely disruptive however we're in a period where wealth around or private standards of living around the world are rising probably faster than any time in human history in China 600 million people have come out of poverty in the last 20 years in Africa life expectancies increasing really rapidly in India and Brazil their challenges that most countries face are not ones of technology they're of miss governance and that's the real cost for most of humanity the technology that we have now allows many countries to move increase their standard of living much much faster than if they had to invent it for themselves right they can make use of that medicine they can make use of those machines there's challenges of that there's property rights as you said who owns the machines who owns the patents on the medicine but the potential to increase human prosperity is enormous and I don't think there's any sense that we'll run out of demand poor people are just like rich people except they don't have money when they get richer they'll want the same stuff right we have no evidence that people run out of ability to consume or desire additional consumption so I'm not that part doesn't concern and then could only Fonda thank you thank you for the fascinating talk you said a couple of things that I think are very interesting but also to me open up a very important question on the one hand the you said and I totally agree that it's very difficult to predict the jobs of the future and at the same time that the best the most effective policy that we can implement to allow people to adapt to new technologies and to benefit from them is education but then what do we teach how do we prepare young workers to a world that we have no idea how we look like well it's it's a good question but I think it's it's comes down to specific versus general skills I don't think we need to teach people Java per se or you need to know how to use this arc welder or you know operate Excel the skills that people have that our truly scarce are the problem solving creativity expression they have to be applied to specific domains but those foundational skills are ones that we need to cultivate in everyone from an early age a specialization comes later and it comes with closer to the labor market in the last four or five or six years of your education when you're preparing into the workforce then you apply those general skills to a specific domain and that's when you make those investments that are very specific but I don't think I think that the fundamentals of Education the old reading writing arithmetic those are as or more important than they've ever been that actually people thinking coherently analytically and expressing and reading and writing are as scarce and as important as they have been at any time in history chatting popping room ultimate Amanda thank you I think the the choice and the complementarity and the substitutability between machines and men is very endogenous now and it's evolution is also very endogenous I mean it was a question on this before but I'm taking the example for example of the the chain for producing cars know which is you know the assembling line is still a very labor-intensive activity in a way it's surprising because we also think it's almost you know machine producing is a very automated thing and in fact it's not and the reason why it is not is that you have to produce many different varieties of the same model to pleased consumers and in fact it would be too costly to have machines really being able to develop all those varieties so the reaction of the industry has been to keep of course these complementaries but making labor much more efficient and reorganizing labor right through lean productions and to making labor also the cognitive part of the labor tasks most much larger so this in a way has increased the productivity of labour and reduced its cost and it makes the optional future option of replacing men with machines even less like no because still you have to have a huge investment in making machines very very intelligent in able to produce all these varieties and on the other hand the productivity of labour now is so high that maybe then we will invest much more in the complementarity between men and machines instead of trying to replace it but I think it is an endogenous pattern that comes out on the one hand from the development of technologies and on the other hand from the fact that companies have invested a lot in making labour or organizing labor on the plan making it much more efficient there's an interesting set of points I mean I would say that there's a lot of machinery that's intended to replace labor but doesn't succeed it actually makes labor more valuable in fact most people you're very if you read a magazine for industrial you know for people who run factories it never says here's a machine that will increase your demand for workers they say here's a machine that will you know replace people doing this and that the other thing it makes sense the machines are supposed to reduce our work but historically as we have automated it's changed work and made it more skill demanding so there was decades of debate about computer numerically controlled machines you probably are aware this so the Milwaukee Matic that I put up right there is a whole history of sociology and industrial relations arguing about whether they be skilled labor and that was the intention the intention was to remove human expertise and replace it with machine expertise it turned out that it created a generation of operators who basically had to be computer programmers right it changed to work from physical work to cognitive work and I think a lot of that goes on in the factory the physical work is much easier to automate than the cognitive work and the flex as you said the flexibility is a huge part of that right machines are good at repetitive actions but if you have to do a lot of variety that's too costly to automate it also matters what the nature of demand is right if we were all just happy to have cheaper cars without lots of new features and lots of variety then we could mass-produce them right the Ford Model T famously it only came in one variety in one color um turns out as people got wealthier they wanted more variety and so that created an additional demand and that's something that we tell tend to underestimate how much people demands rise as they get wealthier in in the US if you wanted to earn what a person earned in 1915 a middle-class worker you could do that by working 17 hours a week most people have chosen to pursue a higher standard of living rather than less work right and so our sort of our rising incomes creating additional demand does tend to call forth additional services and products and experiences that often require people to provide them gratitute in ingress a more professor oh thank you thank you very much professor author we're talking about artificial intelligence and I've seen so much human intelligence in the questions that have been raised so that was a good sign thank you
Contenuti correlati
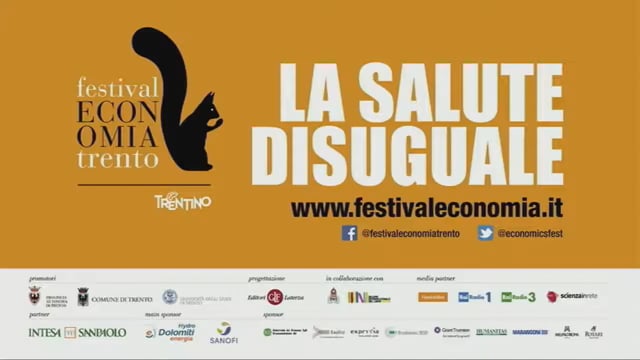
Hyperbolic pension systems
Edizione 2017
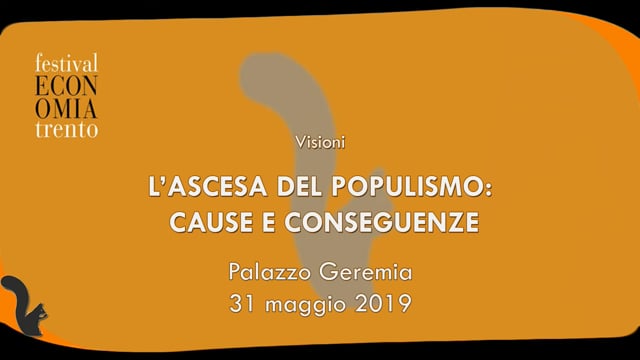
The rise of populism: causes and consequences
Edizione 2019
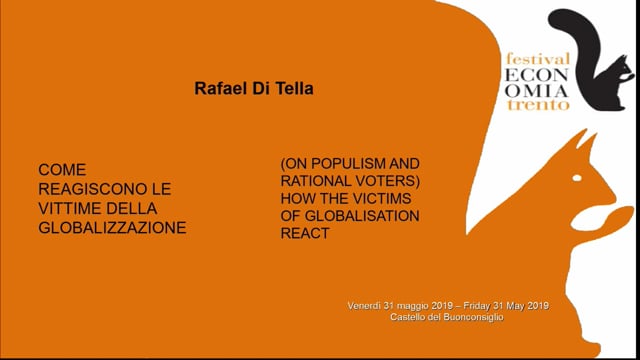
How the victims of globalisation react
Edizione 2019
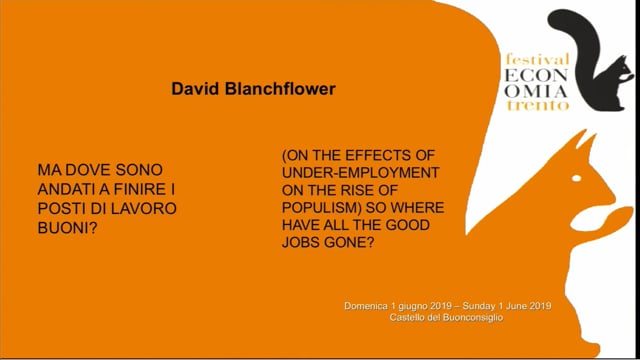
So where have all the good jobs gone?
Edizione 2019
Tematica simile
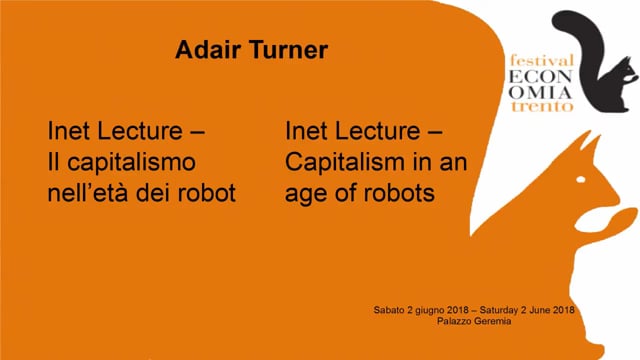
INET Lecture – capitalism in an age of robots
Edizione 2018
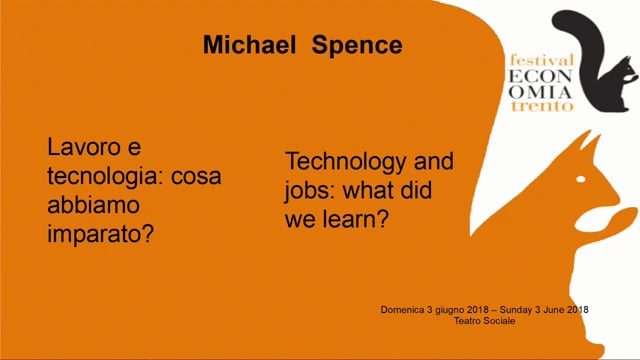
Technology and jobs: what did we learn?
Edizione 2018
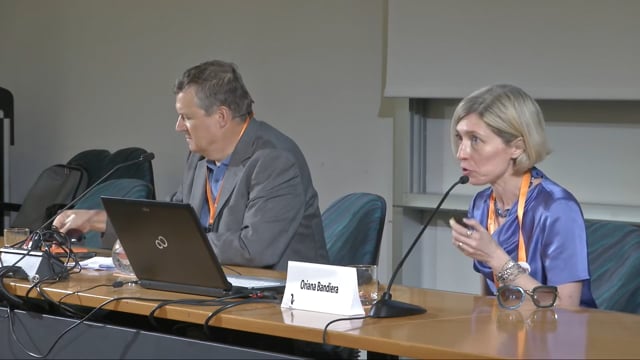
Lavoro, competenze e povertà
Edizione 2018